
#Pump it up how to#
With doing this, we understood the which model approach is true for this data and how to set our parameters for models. To simplify this problem, firstly we collected functional and functional but needs repair wells together and found the best model for binary class. So, we have to find the balanced values for each label in confusion matrix. Our data has highly imbalanced three target labels and all three of them are important to predict as true.
#Pump it up trial#
Because, for the first modeling trial as a baseline with simple logistic regression our model gave 0.83 roc-auc score for binary class. This cleaning process took too much time but at the end, we understood the importance of data cleaning again. For feature engineering, we created new columns for some features and categorized them again manually. So, we dropped some columns which contains same information, converted null and missing values to mean or collected them in unknown category. Lastly, some columns has discrete values. There are spelling mistakes in some columns which creates high unique values. Generally, features are categorical and some of them has more than 2000 unique values. Also, there are many null, zero and missing values. These columns cause multi-collinearity in model. Because, it contains lots of columns which has same information. Mainly, there are two challenges in this data. Train set contains 59400 water points data with 40 features. With given training set and labels set, competitors are wanted to build predictive model and apply it to test set to determine status of the wells and submit. Basically, there are 4 different datasets submission format, training set, test set and train labels set which contains status of wells. Our aim in this project to build a model which predicts the functionality of water points. Water points were divided in three classes as functional, non-functional or functional but needs repair by water ministry. So, I have an interest this type of problems. Even, I have two close friends who live in Tanzania and always tell me about the water shortage of this country.

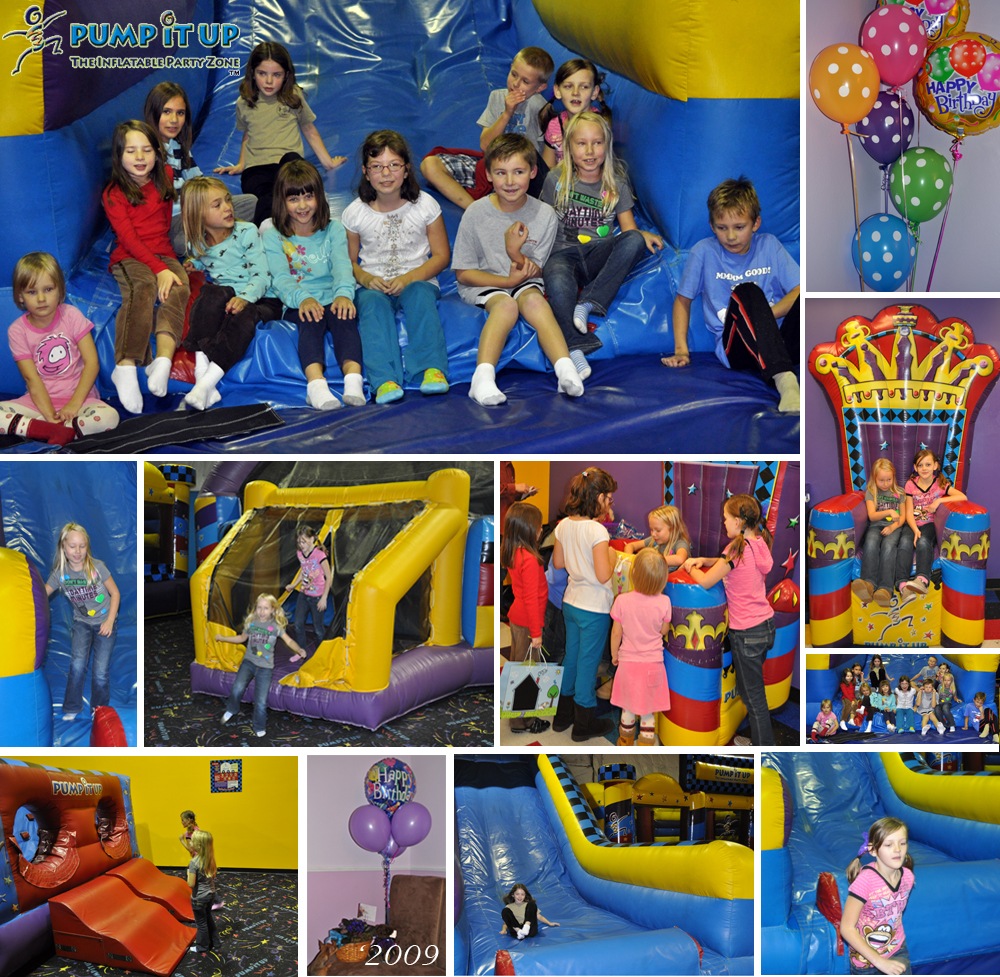
I have worked in NGO for many years to help people. The reason for choosing this project is my interest in solving the main problems that concern humanity.

As a Module 3 project of Flatiron School Data Science Bootcamp, I worked on this problem with Mark Subra. The Tanzanian Water Ministry agreed with Taarifa and they begun a competition by DrivenData to solve this problem with improving clean water sources. 25 million of this population have lack access to clean water, 40 million people also have a lack access to improved sanitation. Tanzania is the largest country of East-Africa with 59 million population. For some areas in the world, to find clean water, pump this water up and transport this water to people are really difficult processes. Despite the unbelievable development of technology, simple basic needs such as access to clean drinking water are still one of the most important problems of human beings.
